Tutorial 1
Security, Privacy, and Trust in Generative AI
Gold Coast Time: Tue 16 Dec 2024 15:30 AEST (UTC+10)
Tokyo Time: Tue 16 Dec 2024 14:30 JST (UTC+9)
â–¶Â Â Abstract
Generative AI systems possess vast capabilities that can significantly enhance creativity and streamline daily business processes. However, alongside these advantages, generative AIs raise critical concerns regarding the protection of individual rights and the potential for psychological or social risks. Issues related to security, privacy, and trust have become priorities for developers seeking to address these challenges. This tutorial provides an overview of potential risks associated with generative AI and explores effective countermeasures, covering topics such as adversarial examples, jailbreaking, machine unlearning, and watermarking techniques.
â–¶Â Â Speaker
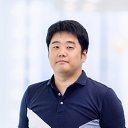
Tsubasa Takahashi
Turing Inc.
Tsubasa Takahashi is a Principal Researcher at Turing Inc. He earned his PhD in Computer Science from the University of Tsukuba in 2014. He has held research positions in industrial laboratories at NEC Corp., LINE Corp., LY Corp., and SB Intuitions Corp., and spent a year as a visiting scholar at Carnegie Mellon University. His research interests include AI security, data privacy, and generative AI systems, with applications in self-driving vehicles.
Tutorial 2
Fairness in graph data analysis
Gold Coast Time: Tue 16 Dec 2024 16:30 AEST (UTC+10)
Tokyo Time: Tue 16 Dec 2024 15:30 JST (UTC+9)
â–¶Â Â Abstract
Fairness in data science is a critical issue in various applications and has become one of the hot topics in research. In this tutorial, I will introduce the topic of fairness in graph data analysis. I will explore the types of biases present in graph data, review existing research, and conclude with a discussion of open problems in this area.
â–¶Â Â Speaker
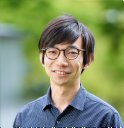
Yuya Sasaki
Osaka University
Yuya Sasaki is an associate professor at Osaka University, Japan. His research focuses on spatio-temporal/graph data management/analysis, trustworthy AIs, and applied data science to other fields such as chemical and medical science.
Tutorial 3
Smart Agriculture Through a Data-Centric Lens: From Human Annotation to Automated Solutions
Gold Coast Time: Wed 17 Dec 2024 15:30 AEST (UTC+10)
Tokyo Time: Wed 17 Dec 2024 14:30 JST (UTC+9)
â–¶Â Â Abstract
With the rapid advancement of digital agriculture, data-driven solutions have become increasingly essential in tackling complex agricultural challenges. Data-driven approaches like machine learning require high-quality, labelled data to train robust and accurate algorithms. To support these efforts, the Global Wheat project has introduced two transformative datasets: Global Wheat Head Detection (GWHD) and Global Wheat Full Semantic Segmentation (GWFSS). The GWHD dataset is pivotal for precise wheat head detection, while the GWFSS dataset enables detailed segmentation within intricate wheat canopies, capturing components like leaves, stems, and inflorescences. These datasets highlight the critical role of expertly curated data in powering effective AI solutions. While human-annotated data is invaluable for training models, the process is often labour-intensive, costly, and challenging to scale. Addressing these issues, the EasyDAM series offers an innovative, automated approach to data annotation through generative AI, reducing the reliance on human labelling in detection tasks. EasyDAM complements initiatives like Global Wheat by providing a generative AI solution for self-annotation, addressing the domain gap between crop types. This approach allows a model trained on one crop to adapt seamlessly to another with minimal labelled data, enhancing adaptability and reducing the burden of data annotation. Together, this talk will explore the construction, outcomes, and future potential of these resources and techniques, offering insights into how they are driving the next generation of data-driven solutions in agriculture.
While human-annotated data is invaluable for training models, the process is often labour-intensive, costly, and challenging to scale. Addressing these issues, the EasyDAM series offers an innovative, automated approach to data annotation through generative AI, reducing the reliance on human labelling in detection tasks. EasyDAM complements initiatives like Global Wheat by providing a generative AI solution for self-annotation, addressing the domain gap between crop types. This approach allows a model trained on one crop to adapt seamlessly to another with minimal labelled data, enhancing adaptability and reducing the burden of data annotation. Together, this talk will explore the construction, outcomes, and future potential of these resources and techniques, offering insights into how they are driving the next generation of data-driven solutions in agriculture.
â–¶Â Â Speaker
Wei Guo is currently an Associate Professor and PI at Laboratory of Field Phenomics, Graduate School of Agricultural and Life Sciences, the University of Tokyo, Japan. Trained as an engineer in computer science/informatics in China and Japan, he received his Ph.D. in agriculture majoring in agro-informatics at the University of Tokyo in 2014. He established the “Laboratory of Field Phenomics”, the first plant phenomics laboratory in Japan, as a core member in 2017. His research focuses on field-based phenotyping using advanced sensing platforms and technologies such as drones and ground robots, image processing, and machine learning approaches. In 2020, Guo was the recipient of the Young Researcher’s Award from the Japanese Society of Agricultural Informatics. Guo is also the senior editor of “Plant Phenomics”
Zijian Wang is a research fellow at the University of Queensland, Australia. His research area mainly on model generalisation in computer vision. His work has been published in esteemed conferences and journals such as NeurIPS, ICML, ICLR, ICCV, MM and TPAMI. Zijian is also widely engaged in cross-disciplinary research projects spanning fields from smart manufacturing to plant phenotyping.
Tutorial 4
Applications of Shapley Values in Data Management and Machine Learning
Gold Coast Time: Tue 17 Dec 2024 16:30 AEST (UTC+10)
Tokyo Time: Tue 17 Dec 2024 15:30 JST (UTC+9)
â–¶Â Â Abstract
The Shapley value offers a robust framework for fairly distributing contributions among participants in cooperative settings. We explore its applications in two critical domains: data management and machine learning. In data management, Shapley values can clarify the contributions of relevant data tuples and improve response accuracy in query answering. Moreover, Shapley values can evaluate the contribution of individual data points on model performance in data valuation. In machine learning, Shapley values provide insights into feature importance and enhance the transparency of decision-making processes. This tutorial aims to deliver a comprehensive understanding of how Shapley values can be leveraged to optimize data management practices and enhance the interpretability of machine learning models.
â–¶Â Â Speaker
Jinfei Liu is currently a 100-Talents Young Professor at Zhejiang University. His research interests include data market, data privacy and security, and data queries. He has published more than 40 papers in premier journals and conferences including SIGMOD, VLDB, ICDE, CCS, TKDE, and VLDBJ and received a best paper award. He has served as a PC member for several top conferences including SIGMOD, VLDB, ICDE, CCS, and KDD.
Jiayao Zhang is currently a 4th year Ph.D. student at Zhejiang University. Her research interests include data markets and data management. She has published a couple of papers in premier conferences including SIGMOD, VLDB, ICDE, and KDD.
Junyuan Pang is currently a 1st year Ph.D. student at Zhejiang University. His research interests include data markets and data management. He has published a couple of papers in premier conferences including SIGMOD, VLDB, and CCS.